IN TODAY'S SIGNAL |
Read time: 4 min 24 sec |
🎖️ Top News
📌 CodeRabbit
⚡️ Trending Signals
📌 NVIDIA
🧠 Top Papers
|
|
|
|
If you're enjoying AlphaSignal please forward this email to a colleague.
It helps us keep this content free. |
|
|
|
TOP NEWS |
OpenAI |
OpenAI announces SearchGPT: An AI search feature that give you fast answers with clear and relevant sources |
⇧ 5519 Likes |
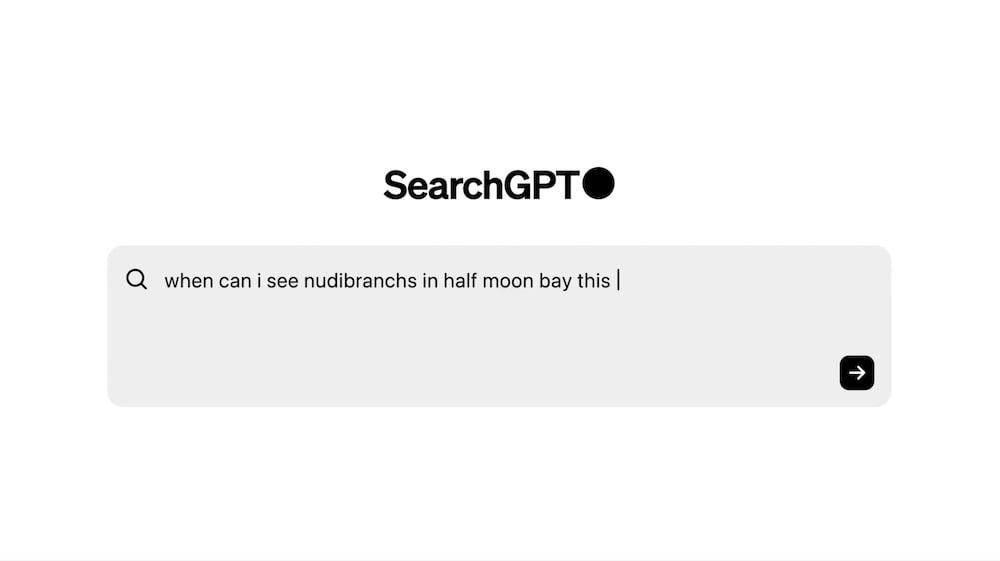 |
What's New |
OpenAI has introduced SearchGPT, an AI-powered search engine prototype. This new tool integrates real-time web information with the conversational capabilities of GPT-4 models, providing fast and relevant answers with clear source links.
How SearchGPT Works
SearchGPT allows users to input queries into a large textbox, which organizes and summarizes findings. It presents short descriptions followed by attribution links, enhancing the traditional search experience by enabling users to ask follow-up questions and explore additional results via a sidebar.
Partnerships and Publisher Collaboration
OpenAI developed SearchGPT in collaboration with publishers like News Corp, The Atlantic, and Vox Media. These partnerships ensure accurate attribution and linking to original sources. Publishers can manage their content appearance in SearchGPT and opt out of generative AI training while still appearing in search results.
User Engagement and Real-Time Capabilities
SearchGPT's real-time information access helps users find relevant answers quickly. The tool supports conversational queries, building context with each question, which is an improvement over traditional search engines that provide a list of links.
Key Metrics and Availability
Initially, 10,000 test users will access SearchGPT, which uses direct content feeds and third-party partners for building search results. OpenAI emphasizes ongoing improvements, acknowledging potential inaccuracies. Users can join a waitlist to try the prototype.
Strategic and Market Implications
OpenAI's launch of SearchGPT challenges Google's search dominance. This move addresses publisher concerns about AI-driven search tools. OpenAI aims to drive traffic to publisher sites while ensuring high-quality information through SearchGPT.
Key Features
- Real-time web information integration
- Conversational query support
- Collaboration with major publishers
- Clear source attribution and linking
- Availability to 10,000 initial test users
|
|
JOIN THE WAITLIST |
|
|
|
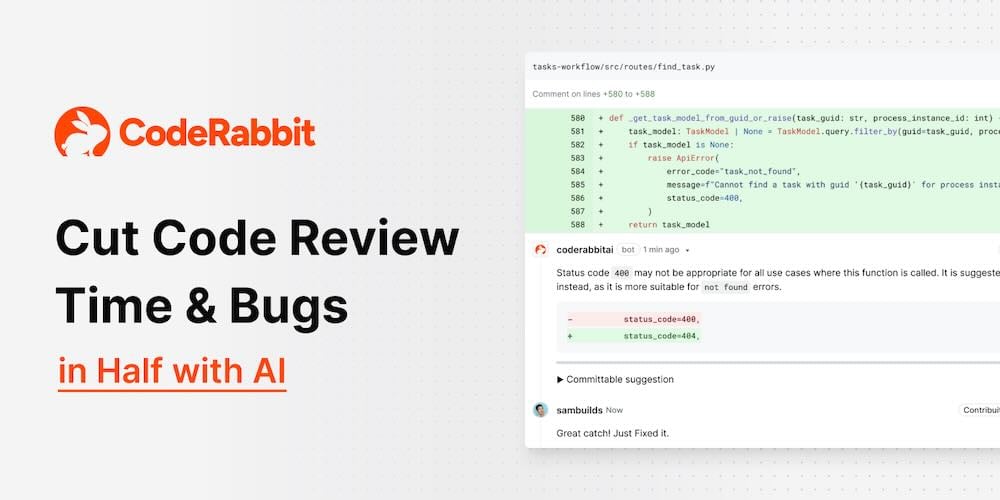 |
CodeRabbit: Merge Code 10x faster with AI-driven code reviews |
With CodeRabbit, you get:
-
Codebase-aware, line-by-line reviews with 1-click fixes.
-
Smart real-time chat for advice, code generation, and issue creation directly from review comments.
-
Comprehensive pull request summaries and sequence diagrams of changes.
Most installed AI app on GitHub and GitLab marketplace, loved by 1000’s of developers.
Enjoy a 7-day trial and access for OSS projects. |
TRY NOW |
partner with us |
|
|
|
TRENDING SIGNALS |
Language Models |
|
⇧ 205 Likes |
|
Open Source |
|
⇧ 1225 Likes |
|
Notebooks |
|
⇧ 242 Likes |
|
Fine-tuning |
|
⇧ 1429 Likes |
|
Open Source |
|
⇧ 1747 Likes |
|
|
|
|
|
|
NVIDIA releases a new way to instantly use and deploy the best models including Llama 3.1 405B, 70B, and 8B |
Their AI Foundry lets you create custom "supermodels" tailored to your needs and train them with proprietary data as well as synthetic data generated from Llama 3.1 405B.
It can handle, data curation, synthetic data generation, fine-tuning with proprietary data, accurate response retrieval, comprehensive evaluation and deployment. |
Try it now ↗️ |
|
|
|
TOP PAPERS |
Video Generation |
|
⇧ 1528 Likes |
Problem
Reconstructing dynamic scenes from single videos is complex due to the ill-posed nature of the task. Traditional methods are limited as they require templates, function only in nearly static scenes, or cannot track full-sequence 3D motion, which makes them unsuitable for complex, moving scenes.
Solution
This approach uses SE(3) motion bases to model motion as a combination of base movements. It integrates data-driven priors like depth maps and 2D motion tracks into a unified scene representation, enhancing consistency and accuracy.
Results
The technique sets a new standard in 3D/2D motion tracking and novel view synthesis. It lowers 3D tracking error to 0.082 EPE and raises 3D tracking accuracy (within 5cm) to 43.0% on the iPhone dataset.
|
|
Language Models |
|
⇧ 1411 Likes |
Problem
Modern AI requires foundation models that integrate multilinguality, coding, reasoning, and tool usage. Existing models, while advanced, do not fully integrate these capabilities with high performance across various tasks.
Solution
Llama 3, a dense Transformer model with 405B parameters and a 128K token context window, addresses this need. It was pre-trained on a 15T token multilingual corpus using 3.8 × 10^25 FLOPs, significantly outscaling previous models. Llama 3 supports extensive multilingual capabilities and integrates coding, reasoning, and tool usage more seamlessly.
Results
Llama 3 matches GPT-4's performance across numerous benchmarks. On tasks like MMLU and IFEval, Llama 3.1 405B scores 87.3% and 88.6%, respectively, showing competitiveness with or superiority to other models. Smaller versions also outperform comparable models, making Llama 3.1 a leading option across size scales. |
|
KAN |
|
⇧ 685 Likes |
Problem
Comparisons between Kolmogorov-Arnold Networks (KAN) and Multi-Layer Perceptrons (MLP) are unfair due to differing parameters and FLOPs.
Solution
This study controls parameters and FLOPs to fairly compare KAN and MLP across tasks like machine learning, computer vision, NLP, audio processing, and symbolic formula representation. B-spline activation's impact is explored.
Results
MLP outperformed KAN in machine learning (86.16% vs. 85.96%), computer vision (85.88% vs. 77.88%), NLP (80.45% vs. 79.95%), and audio processing (17.74% vs. 15.49%). KAN excelled only in symbolic formula representation (1.2e-3 RMSE vs. 7.4e-3). Access the code here. |
|
|
|
|
|
|
|